Research data storage
As a researcher at Lund University, you are obligated to handle your research data in a way that protects individuals, society, and Lund University from harm. To help you achieve this, you are offered a storage solution that is free of charge. You are also provided support on how to assign a security classification to your research data and thereby select the proper level of security for your data.
On this page
- Research data folders
- Security classification of research data
- Selecting the proper security level
- Store and handle sensitive data free of charge
Research data folders
As a researcher or project manager you can now store your research data in a research data storage solution that is free of charge. The new solution offers research data folders with improved back up and greater control and authorisation management options. You order your research data folders in Lucat.
Ordering research data folders for the first time
If you are ordering research data folders for the first time, you need to:
- Read the information on security classification of research data to ensure you choose the right storage and handling solution.
- Go to the webpage with gathered important information on how to order, get started with and administer research data folders in Lucat.
- Place your order for research data folders in Lucat.
- Security classification of research data
- Get started with your research data folders
- Order research data folders in Lucat
Security classification of research data
When you request a research data folder, you will need to assign a security classification to your data. This means that you need to select one of four security levels based on the severity of the consequences for the individual, society or university if your research data is disclosed, corrupted or made inaccessible.
- Data disclosure means that unauthorised persons gain access to information they should not have.
- Data corruption means that data is altered or manipulated so that it is no longer accurate or reliable. For example, the results of a research study are changed.
- Making data inaccessible means that it can no longer be accessed by those who need it. For example, research data is lost due to a system failure.
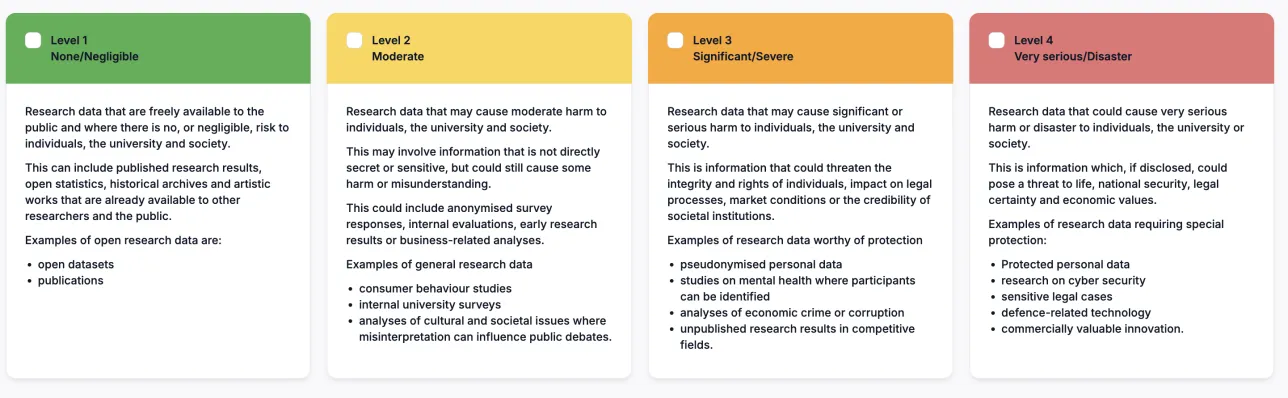
Selecting the proper security level
When selecting security level for your research data, it is important to always consider the specific context. The same dataset can have different security levels depending on the context in which it is used. Geographical coordinates may be completely harmless in a study on bird migration, but sensitive in a study on protected species, where the coordinates may reveal specific locations of endangered species.
The highest security level is for data where a leak could result in very serious harm or catastrophic consequences, such as direct danger to life and safety.
Examples of level 4 data
- Sensitive personal data that allows for direct identification of single individuals (such as medical records with personal identification numbers)
- Video interviews on religion or political activity
- Critical infrastructure, defence or biological threat data
- Quantum encryption and laser technology
- Dual-use products.
In-depth example
- A researcher is working with a dataset containing names, addresses and social security numbers of individuals with protected identities. A leak could expose these individuals to serious danger or threat, and cause great anxiety and psychological stress to the individuals concerned. The university risks legal consequences and a serious loss of trust. The researcher therefore classifies the data as Level 4.
- A is a researcher researching dual-use products, i.e. those that can be used for both civilian and military purposes. The researcher has data on advanced chemical processes that can be used for medical purposes, but also to create biological weapons, which could have catastrophic consequences. Therefore the researcher classifies the data as Level 4.
Here, a leak may result in significant or serious harm to single individuals, the society or Lund University.
Examples of level 3 data
- Sensitive personal data that may allow for indirect identification of single individuals, e.g., by the amount of data collected (pseudonymised data).
- Studies on minors or other vulnerable groups, even when the data are not considered sensitive.
- Politically sensitive topics, such as corruption networks or organised crime.
- Unpublished research results with commercial value.
In-depth example
A researcher has pseudonymised interview data with young people who talk openly about their mental health problems. The dataset is linked to a code key that enables identification. If this is revealed, young people may face social stigmatisation, poor mental health and other personal problems. The university may also face criticism for lack of data security. The researcher therefore classifies the data as level 3.
Here the risk of harm from leaking is moderate. For example, disclosure of data from a survey on eating habits may lead to some concern among participants, especially if the data may be combined with other information that allows for indirect identification of participants. Lund University risks some reputational damage and reduced participation in future studies.
Examples of level 2 data
- Anonymised sensitive personal data, i.e., data that do not allow for either direct or indirect identification of single individuals.
- Survey responses on non-sensitive questions but with indirect identifiability.
- Internal studies with risk of reputational impact.
- Non-sensitive infrastructures and historical materials.
In-depth example
A researcher collects air and soil samples in the vicinity of a large industrial area to analyse heavy metal levels and other environmental toxins. Although no personal data is involved, dissemination of the results before they are scientifically validated may lead to erroneous conclusions in the media or public concern in society. It may also affect industry's relations with the environment or give rise to speculation about environmental impacts. The researcher therefore classifies the data as Level 2.
This applies to information where the risk is non-existent or negligible. A researcher publishes datasets in open repositories where the information is of public interest and fully anonymised. The risk of disclosure is minimal, as the data is already intended for public access and use.
Examples of Level 1 data
- Published datasets in open repositories.
- Metadata.
- Material from open sources.
- Public websites.
In-depth example
A researcher publishes datasets from climate research in open repositories, which include fully anonymised and harmless measurement data from weather stations. As the data is already intended for public access, the risk of negative consequences is non-existent. The researcher therefore classifies the data as Level 1.
Store and handle research data worth protecting free of charge
If your data classifies as level three or four, you currently need to rely on one of the secure systems offered by Lund University; LUSEC or COSMOS-SENS. However, work is ongoing to develop a simple and uniform solution. Meanwhile, the cost of using LUSEC and COSMOS-SENS has been taken over by central functions and there will be no charges to researchers.
Contact
You can get advice and guidance on research data management from the University's joint research data support team. The support team includes:
- Faculty Data Stewards
- Faculty Library Research Support
- Archivists.
Submit your question (web form)
Email: support [at] researchdata [dot] lu [dot] se (support[at]researchdata[dot]lu[dot]se)
Anonymised or pseudonymised personal data?
Anonymised personal data is data from which all identifying information has been removed and which cannot be traced back to individuals in any way. Pseudonymised personal data means that identifying information has been removed, but it is still possible to trace the information back to an individual, for example through a code key or through other variables.
Want to learn more about personal data in research?
In the Competence Portal you can take the Personal data in Research course. The course is online and takes about 30 minutes to complete.